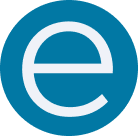
epocrates
Podcast Recap | Academic Medicine Podcast: Using AI tools to ease the workload burden on faculty
December 28, 2023

Experts Gustavo Patino, assistant editor at Academic Medicine and associate dean for undergraduate medical education at Western Michigan University Homer Stryker M.D. School of Medicine, Christy Boscardin and Brian Gin, authors of “ChatGPT and Generative Artificial Intelligence for Medical Education: Potential Impact and Opportunity,” and Marc Triola, author of “Artificial Intelligence Screening of Medical School Applications: Development and Validation of a Machine-Learning Algorithm” dissect the opportunities surrounding artificial intelligence (AI) in medical education, but also raise concerns related to bias, data privacy, and ethics, painting a nuanced picture of the future it holds. Here are our takeaways from the podcast.
Podcast length: 46 min.
5 Key Takeaways
1. Two of the biggest potential applications of tools like ChatGPT in academic medicine include writing assessment items that can be used in teaching activities, and scoring assignments.
According to Boscardin, for faculty with limited access to real case clinical scenarios, generative AI tools could be helpful in the creation of clinical scenarios used for learning cases or as part of assessment prompts. Additionally, AI could help generate actual assessment items. Boscardin has already seen surprising outcomes when using AI to create board style exam questions.
Scoring, Boscardin explains, is another potential application of AI as exams move toward more open-ended response prompts and open-ended questions. Open-ended items are not only time-consuming but also could be prone to rater bias and reliability challenges. Boscardin believes a tool like ChatGPT could help instructors review and score some of the open-ended response items or assignments that require text analysis using a scoring rubric.
2. Medical schools across the country are faced with a rising number of medical school and residency program applications, but few faculty are available to screen them. A team at NYU recently explored how AI can be used to create a more holistic and efficient approach to reviewing these applications.
In 2019, Triola and his colleagues at NYU were faced with an enourmous challenge following the announcement of full tuition scholarships for every student who enrolled. The number of people who applied to NYU’s medical school increased from approximately 6,500 per year to just under 10,000 per year.
Triola and his team began to explore how AI could help to streamline and optimize the medical school application process. They were fortunate to have many years of high-density digital data on the applications, as well as early screenings they had previously used to determine whether or not applicants would be offered an interview. They used this digital data to create, train, and validate an AI/machine learning (AI/ML) model to predict the way that human faculty might have screened an application.
The team spent two years conducting a real-world randomized, prospective trial which compared the outcome of faculty screenings to AI/ML screenings. They wanted to ensure the absence of biases in their model, so they looked carefully at the outcomes, specifically the recommendations for interviews or rejections among all of the students, including those who are underrepresented or historically excluded in medicine by gender, etc.
Ultimately, they were thrilled with the results, which demonstrated that with a large amount of data, you can train a model that accurately replicates the decision-making process of human faculty screeners. Today, this AI-run system saves Triola and his team nearly 6,000 faculty hours per year by quickly screening applications in a fair, transparent, and consistent way.
3. Faculty and students should be aware that using a public AI system like ChatGPT on the web ultimately means you are contributing your data to another system in a manner that is outside of your control.
Triola warned that protected health information, including both medical student data and research data, should not be placed into public AI systems, noting that at the beginning of the NYU trial, his team acquired a unique HIPAA-compliant version of ChatGPT 3.5 and 4 that could be used for experimentation.
According to Triola, protecting health information will continue to be a big challenge as companies begin adding AI to Gmail, Google Docs, Microsoft Word, PowerPoint, and more. Faculty, students, and residents may even be using AI while not being fully aware. Medical schools must institute community policies and guidance to govern the appropriate use of these systems, including defining what constitutes data privacy.
In health care, AI may be used to communicate with patients, students, and faculty and AI systems must know when to deliver these communications and when to delegate the task to a person, even if the AI may be better than the person at delivering that information.
4. When used in health care spaces, AI models—whether generative or predictive—should be evaluated based on trustworthiness.
Gin explains, “trust is such a key element of how we operate in medicine, [including] how it really forms our ability to do work and take care of patients.”
Further, he stresses that the trustworthiness criteria of generative AI systems must be defined, similar to that used to entrust assessments and learners, or to trust a trainee to perform a clinical task. Gin suggests that the same questions should be asked of AI models.
Frameworks like Meyer, et. al., which include criterion used to determine the trustworthiness of an individual, may provide a suitable guide for AI systems. AI systems should be evaluated based on the following:
- Knowledge and experience required to perform the task, including applicability to respond to the questions it’s asked with valid responses
- Benevolence and integrity, including defining responsibility for various outcomes
- Ability to act in an honest and ethical manner without algorithmic biases related to gender, demographics, etc.
5. The medical community should be involved in establishing reporting standards with the use of AI and AI analytic tools like ChatGPT, including improved transparency and appropriate attribution of these tools.
Lack of attribution in generative AI outputs is among the biggest limitations of the current technology. As AI tools improve, citing primary sources of data or information used to create that output will be critical.
When machine learning and large language models are used in medical education, greater transparency will be required, including documenting the steps in the analytic process, providing rationale for the chosen classifiers and analytics used, validating the algorithms used in the training data, defining the decisions made in each process, and justifying the statistical modeling.
Any views, thoughts, and opinions expressed in this podcast recap are solely that of the host and guests and do not reflect the views, opinions, policies, or position of epocrates and athenahealth.
Source:
AM Rounds. (2023, Dec. 13). Academic Medicine Podcast. Do What You Do Better: Using AI Tools to Ease the Workload Burden on Faculty. https://academicmedicineblog.org/category/audio/.
TRENDING THIS WEEK